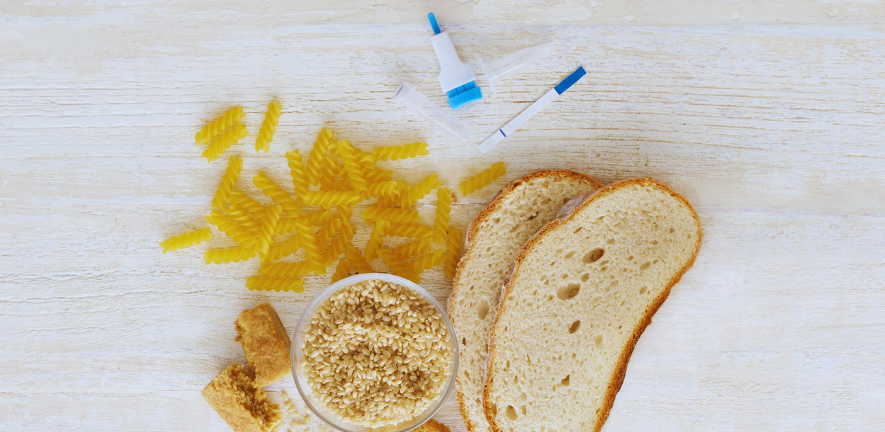
Submitted by Livia Harriman on Tue, 25/03/2025 - 12:19
A pioneering artificial intelligence (AI) tool developed in Cambridge has demonstrated its potential to revolutionize the diagnosis of coeliac disease, significantly accelerating the process and alleviating pressure on healthcare systems.
The groundbreaking study, published in the New England Journal of Medicine, showcases how AI can automate the analysis of biopsy images with high accuracy, expediting patient diagnoses and improving access to specialist care.
Coeliac disease affects approximately 1 in 100 people worldwide, causing symptoms such as stomach cramps, diarrhea, skin rashes, fatigue, and anaemia. Diagnosis requires skilled pathologists to analyze intestinal biopsy samples, which can be time-consuming and sometimes inconclusive.
"This is the first time AI has been shown to diagnose as accurately as an experienced pathologist whether an individual has coeliac disease or not," said Dr Florian Jaeckle from the University of Cambridge's Department of Pathology, the study's first author. "Our next step is to test the algorithm in a much larger clinical sample, moving us closer to making this tool available for widespread use, including within the NHS."
The AI model was trained using 4,000 biopsy images from five NHS hospitals, enabling it to distinguish between healthy samples and those with coeliac disease. When tested on an additional 650 biopsies, the AI achieved an impressive accuracy rate of over 97%, surpassing the consistency levels of human pathologists.
Professor Liz Soilleux, study lead and Honorary Consultant Pathologist at Cambridge University Hospitals NHS Foundation Trust, emphasized improving diagnostic timelines: "Coeliac disease can take years to diagnose, leaving many patients without answers. AI offers the potential to speed up this process, helping patients receive a definitive diagnosis more quickly while reducing NHS waiting times."
A Patient's Perspective
Liz Cox, 80, from Linton, was diagnosed with coeliac disease in her 50s after experiencing unexplained symptoms for almost three decades. A chance remark from a friend about her weight loss prompted her to seek further testing, leading to a biopsy-confirmed diagnosis. "Anything that makes the system quicker must be good," said Liz. "Once you've been diagnosed, you know what to do—avoid gluten. The sooner people get that clarity, the better."
As secretary of a Coeliac UK support group for over twenty years, Liz has witnessed firsthand how long and frustrating the diagnostic journey can be for many individuals. She hopes AI-driven diagnostics will offer a much-needed solution to those still searching for answers.
Towards Real-World Application
The research team is now focused on ensuring that AI-aided diagnoses are explainable and transparent to clinicians and patients, addressing concerns about how AI reaches its conclusions. "Creating understandable AI diagnoses is key to gaining regulatory approval and trust for use in clinical settings," noted Professor Soilleux.
The study was funded by the National Institute for Health and Care Research, with commercial development led by Lyzeum Ltd, a University of Cambridge spinout company.
About the Research
This study was conducted by researchers at the University of Cambridge in collaboration with multiple NHS hospitals. The AI tool is designed to be adaptable across diverse healthcare environments, including regions with limited access to specialist pathologists.
About Coeliac Disease
Coeliac disease is an autoimmune condition triggered by gluten consumption. Left undiagnosed, it can lead to severe complications, including malnutrition and long-term health issues. The only treatment is a strict gluten-free diet.
About Lyzeum Ltd
Lyzeum Ltd is a spin-out company from the University of Cambridge, dedicated to commercializing AI-driven diagnostic tools for improved patient outcomes.
Read a special feature:
Using AI to diagnose coeliac disease
Read the Guardian news article here:
Interpretable Machine Learning-based Detection of Coeliac Disease